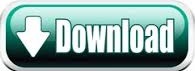
The logistic map, despite being quite simple on the surface, gives us a surprisingly good prediction of observed population dynamics in the real world. That’s where the (1 - x n) comes from – it reflects the fact that there’s only so many bunnies that can live on one hill before they simply become too successful for their own good. But if there are too many bunnies, the food will run out, and some will be forced to leave (or starve). The more bunnies we have, the more there are to reproduce, so we multiply the reproduction rate by the number of bunnies in the current population, x n. Let’s suppose we’re modeling how a colony of rabbits changes over time: then the logistic map tells us that how many bunnies we have tomorrow depends on how many we have today together with the reproduction rate of the population – how fast they, ahem, make new bunnies. Remember, the logistic map started as a way to model population dynamics, and that’s a pretty good way of thinking about what’s going on here.

Image credit: IFLScienceĪnd if we increase r up to 3.5 we have Now the values of x are bouncing around between four different values. On the other hand, if we set r = 2.5 we get We've only changed r by a small amount, but now the values of x very quickly approach the value 0.6. You can see them jump about, known as oscillation, between about 0.6 and 0.7. Then the map will give us Each subsequent value of x is given by applying the recurrence relation. Let’s choose as our starting point x 0 = 1/2 (we’ll always choose a value between zero and one for our starting point, and 1/2 is nice and central) and we’ll set the value of r to be 3.
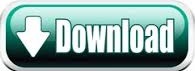